Reflections, Summer 2013
Back to ICSI Gazette, Summer 2013
by Roberto Pieraccini, CEO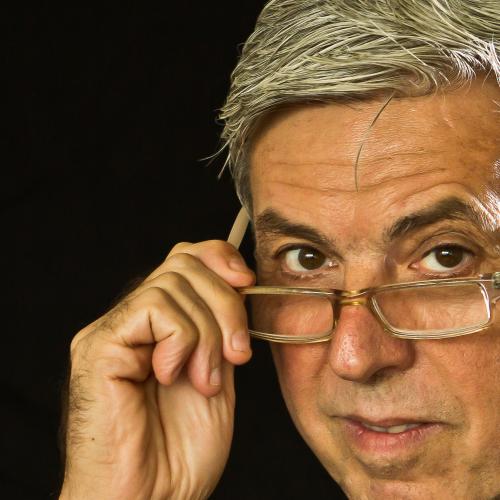
Of the many words that characterize the last few years of technical innovation, three stand out: “big,” as in big data; “many,” as in the use of many people to help make sense of big data; and “deep,” as in deep learning. What is big about big data is the notion that, in an unprecedented way, we have access to amounts of data so large that we are facing not only new and seemingly insurmountable problems, but also great, or rather big, new opportunities for a deeper understanding of the phenomena represented by data. The problems derive from the sheer size of the ever-growing available data: simply storing, handling, and analyzing massive amounts of data is a problem in itself. The opportunities, however, are endless, and they promise to be epochal. If we can process and extract the appropriate knowledge from these large amounts of data, we can answer the most important unanswered questions about our world and create new tools that would benefit society at large.
Just look, for instance, at the growth rate of the data that we most frequently access. The numbers are mindboggling: as just one example, YouTube recently reported that 72 hours of video are uploaded to its Web site every minute! That’s big data. Finding a video, unless it is properly tagged with text, is like finding a needle in a haystack. While searching huge amounts of text can be done in real time — and we do this every time we run a Web search — searching for visual concepts within an enormous and ever-growing corpus of video and audio may be beyond our current computational capability. Finding out how to do this with what we have is a difficult problem to solve, but the solution would give us the unprecedented opportunity to take advantage of all the data, video, and audio - and not just text — that is available on the Web for deeper understanding and deeper searches.
In order for machines to learn how to use big data, we need some level of supervision from humans, known as the annotation process in machine learning. Most machine learning techniques start with a sizable amount of human-annotated data, in which every sample is associated with some form of truth or knowledge representation. Often many non-experts are able to annotate certain types of data, like video or audio. With the advent of crowd-sourcing, for instance with Amazon’s Mechanical Turk, it is possible to automate the whole process: crowd enrollment, data distribution, payment, and management. Machine learning complements the annotation work of crowds and increases its efficiency and accuracy. Data alone, even big data, is not enough for many tasks. The possibility of having big data annotated with the help of machines and crowds in order to come to a deeper understanding of our world is huge. That’s the effort undertaken by ambitious initiatives such as UC Berkeley’s AMPlab, which includes some of ICSI’s affiliated researchers, and by other research initiatives at ICSI.
Genomics is another area that benefits from the availability of big data. The cost of DNA sequencing is predicted to decline at a rate of half every six months, and even though that rate can slow temporarily because of economic forces, it is still a much faster pace than the growth of computational power predicted by Moore’s law. If that holds, the amount of genomic data available either from a single individual or from an entire population is bound to grow disproportionately compared with our ability to process it in a timely manner. That’s the problem, and unless we find ways to speed up the computing power of our machines much faster than Moore’s law, we need to work hard at trying to understand new ways, new algorithms, to manipulate that vast amount of data. But the potential rewards are enormous, including that of finding personalized treatments for cancer that are more effective and more timely than they are presently and that can help increase the chances of patient survival. In other words, we must use BIG data for a DEEP understanding of our world, and MANY are helping toward that goal.